Generative Adversarial Networks for Anonymous Acneic Face Dataset Generation
April 2024
in “
PLoS ONE
”
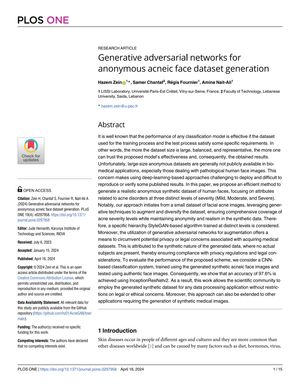
TLDR The method creates realistic, anonymous acne face images for research, achieving 97.6% accuracy in classification.
The paper presents a method to generate a realistic anonymous synthetic dataset of human faces with acne at varying severity levels using a StyleGAN-based algorithm. This approach addresses the lack of large, balanced, and representative datasets in biomedical applications, particularly for pathological human face images. By leveraging generative adversarial networks, the method ensures privacy and legal compliance, as no real subjects are involved. The generated synthetic dataset was used to train a CNN-based classification system, achieving an accuracy of 97.6% with InceptionResNetv2 when tested on authentic face images. This work facilitates the use of synthetic datasets in scientific research without legal or ethical restrictions and can be extended to other medical imaging applications.