Weakly Supervised Learning of Biomedical Information Extraction from Curated Data
January 2016
in “
BMC Bioinformatics
”
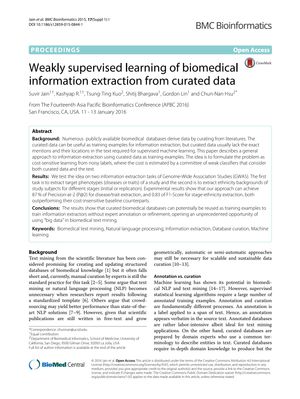
TLDR The method can effectively extract biomedical information without needing expert annotation, performing better than previous models.
In the 2016 paper by Jain et al., the authors introduced a weakly supervised learning method that utilizes curated biomedical databases as a source of training data for information extraction tasks, specifically avoiding the need for expert annotation. They approached the problem as cost-sensitive learning from noisy labels, employing a committee of weak classifiers to estimate costs based on both the curated data and the text itself. The method was applied to extract target phenotypes and ethnicity backgrounds from Genome-Wide Association Studies (GWAS) articles. The results showed that their method achieved a Precision-at-2 of 87% for disease/trait extraction and an F1-Score of 0.83 for stage-ethnicity extraction, surpassing the performance of cost-insensitive baselines. This study demonstrated the potential of reusing curated biomedical databases for training information extraction systems and highlighted the benefits of a cost-sensitive learning approach in biomedical text mining.