Attention Balanced Multi-Dimension Multi-Task Deep Learning for Alopecia Recognition
May 2023
in “
Indian journal of science and technology
”
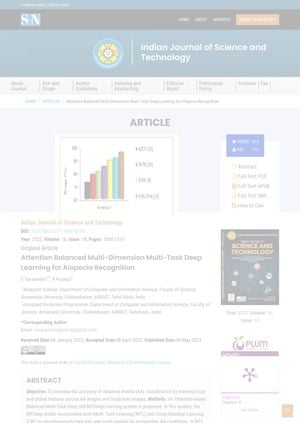
TLDR The new deep learning system can accurately recognize hair loss conditions with a 95.11% success rate.
The study "Attention Balanced Multi-Dimension Multi-Task Deep Learning for Alopecia Recognition" proposes an Attention-based Balanced Multi-Task Deep (AB-MTDeep) learning system to increase the accuracy of Alopecia Areata (AA) classification. This system uses Multi-Task Learning (MTL) and Cross-Residual Learning (CRL) to train hair and scalp images simultaneously for recognizing AA conditions. The model learns both local and global features at multiple scales, which are then classified to recognize AA conditions. The test results show that the AB-MTDeep system achieves an accuracy of 95.11% on hair and scalp image databases, significantly outperforming other classical systems. This suggests that the model could be a promising classifier for AA classification.