Methods of Transfer Learning for Multiclass Hair Disease Categorization
December 2023
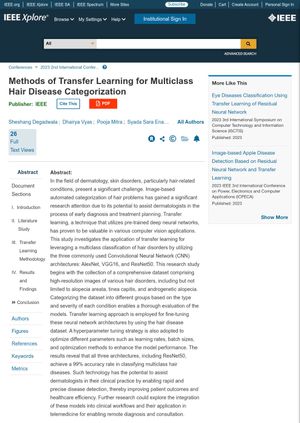
TLDR Transfer learning with three neural network architectures accurately classifies hair diseases.
The study "Methods of Transfer Learning for Multiclass Hair Disease Categorization" investigates the use of transfer learning, a technique that uses pre-trained deep neural networks, for classifying hair disorders. The researchers used three Convolutional Neural Network (CNN) architectures: AlexNet, VGG16, and ResNet50. They collected a comprehensive dataset of high-resolution images of various hair disorders, including alopecia areata, tinea capitis, and androgenetic alopecia, and categorized them based on type and severity. The transfer learning approach was used to fine-tune these neural network architectures using the hair disease dataset, and a hyperparameter tuning strategy was adopted to optimize parameters such as learning rates, batch sizes, and optimization methods. The results showed that all three architectures achieved a 99% accuracy rate in classifying multiclass hair diseases. This technology could potentially assist dermatologists in their clinical practice by enabling rapid and precise disease detection, thereby improving patient outcomes and healthcare efficiency.