Bayesian Measurement-Error-Driven Hidden Markov Regression Model for Calibrating the Effect of Covariates on Multistate Outcomes: Application to Androgenetic Alopecia
September 2018
in “
Statistics in Medicine
”
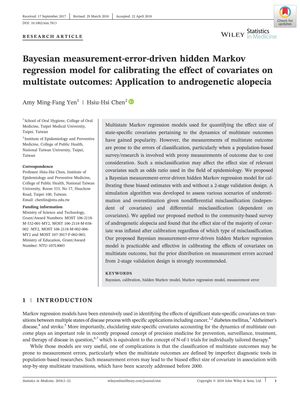
TLDR Model improves accuracy in predicting hair loss effects.
The paper proposes a Bayesian model for calibrating the effect of covariates on multistate outcomes, with an application to androgenetic alopecia. The model addresses the issue of measurement errors in multistate outcomes, which can lead to biased estimates of the effect size of relevant covariates. The study found that after making allowance for the errors of misclassification, the effect of metabolic syndrome on the occurrence of intermediate stage of AGA and on the transition from intermediate to severe state has been inflated from 1.02 to 1.04 for the first transition and from 1.16 to 1.23 for the second transition corresponding to the uncalibrated model and the calibrated model one.