Inferring Spatially Resolved Transcriptomics Data from Whole Slide Images for the Assessment of Colorectal Tumor Metastasis: A Feasibility Study
November 2022
in “
bioRxiv (Cold Spring Harbor Laboratory)
”
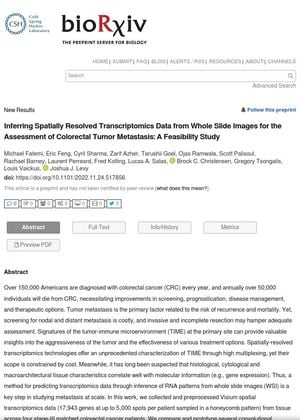
TLDR Using deep learning to predict gene expression from images could help assess colorectal cancer metastasis.
The study "Inferring Spatially Resolved Transcriptomics Data from Whole Slide Images for the Assessment of Colorectal Tumor Metastasis: A Feasibility Study" explores the use of spatially-resolved transcriptomics technologies to study metastasis in colorectal cancer (CRC). The researchers collected and preprocessed Visium spatial transcriptomics data from tissue across four stage-III matched CRC patients. They compared and prototyped several neural networks to predict spatial RNA patterns, hypothesizing that transformer and graph-based approaches would better capture relevant spatial tissue architecture. However, the results showed that the transformer and graph-based approaches did not outperform the convolutional neural network architecture, although they did exhibit optimal performance for relevant disease-associated genes. The study suggests that different neural networks operating on different scales are relevant for capturing distinct disease pathways. The findings add further evidence that deep learning models can accurately predict gene expression in whole slide images, which could be useful for predicting metastasis and other applications.