Peer Review of Computational Drug Repositioning Based on Side-Effects Mined from Social Media
February 2016
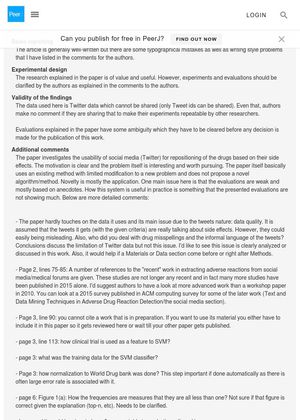
TLDR Reviewers criticized the study for assuming drugs with similar side-effects work the same way and questioned the validity of its findings due to potential biases and data quality issues.
In 2016, a study was conducted to develop a computational method for drug repositioning using side-effects data from social media. The study was critiqued for its assumption that drugs with similar side-effects might share a common mechanism of action. Reviewers also highlighted potential biases and limitations in the experimental design and data collection methods. They questioned the validity of the study's findings, noting that the common side-effects shared by drugs in the study are associated with a large number of drugs, and other researchers have found an inverse correlation between side-effect frequency and the likelihood of two drugs sharing a protein target. The reviewers also criticized the study for its limitations, mainly referring to an unpublished paper, and called for a better description of these limitations. They also pointed out issues with the data used, which was Twitter data, and the ambiguity in the evaluations explained in the paper. They suggested that the paper needed to address the issue of data quality due to the nature of tweets and discuss how they dealt with potential misleading information, drug misspellings, and the informal language of tweets.