An Improved Face Image Restoration Method Based on Denoising Diffusion Probabilistic Models
January 2024
in “
IEEE access
”
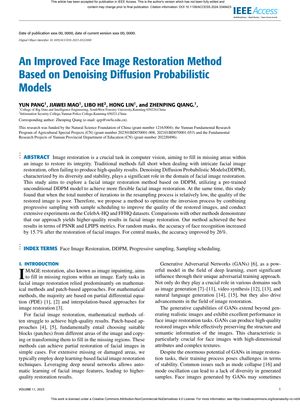
TLDR The new method improves facial image restoration quality and face recognition accuracy.
This study presents an improved facial image restoration method using Denoising Diffusion Probabilistic Models (DDPM). By leveraging a pre-trained unconditional DDPM model, the researchers aimed to enhance the flexibility and quality of facial image restoration. They identified that low iteration counts in the resampling process led to poor image quality and proposed an optimization method combining progressive sampling with sample scheduling. Extensive experiments on the CelebA-HQ and FFHQ datasets showed that their approach outperformed traditional methods, achieving the best results in PSNR and LPIPS metrics. Additionally, the accuracy of face recognition improved by 15.7% for random masks and 26% for central masks after restoration.