DNN-DTIs: Improved Drug-Target Interactions Prediction Using XGBoost Feature Selection and Deep Neural Network
August 2020
in “
bioRxiv (Cold Spring Harbor Laboratory)
”
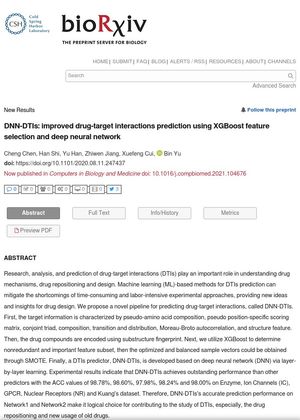
TLDR The DNN-DTIs method accurately predicts drug-target interactions and is useful for drug repositioning.
Three years ago, a novel pipeline for predicting drug-target interactions (DTIs), called DNN-DTIs, was proposed. This method used machine learning to overcome the time-consuming and labor-intensive nature of traditional experimental approaches. The process involved characterizing target information using various methods, encoding drug compounds using substructure fingerprint, utilizing XGBoost to determine nonredundant and important feature subset, and developing a DTIs predictor based on deep neural network (DNN) via layer-by-layer learning. The DNN-DTIs showed superior performance compared to other predictors, with accuracy values of 98.78%, 98.60%, 97.98%, 98.24% and 98.00% on Enzyme, Ion Channels (IC), GPCR, Nuclear Receptors (NR) and Kuang's dataset. This made DNN-DTIs a logical choice for studying DTIs, particularly for drug repositioning and finding new uses for old drugs.