Detecting Shortcut Learning for Fair Medical AI Using Shortcut Testing
July 2023
in “
Nature Communications
”
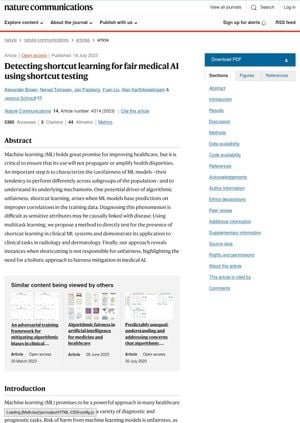
TLDR The ShorT method can detect and help reduce bias in medical AI by identifying shortcut learning.
The study "Detecting shortcut learning for fair medical AI using shortcut testing" investigates the issue of shortcut learning in medical AI, where models may rely on non-causal correlations or overemphasize certain attributes, leading to biased results. The researchers developed a method called Shortcut Testing (ShorT) to identify and mitigate this issue. They used an open-source chest X-ray dataset and trained separate deep learning models, finding that the models do learn to encode age, and their performance varies systematically with age, exhibiting unfairness. The ShorT method was able to detect shortcut learning efficiently. The study also found that even if shortcut learning is not detected, encoding may present intrinsic ethical concerns and potential for misuse. The researchers recommend the removal of the demographic prediction head after training to avoid potential misuse. The study concludes that the ShorT method represents a practically applicable framework for studying and mitigating shortcut learning in clinical ML models.